Atomo’s patient finding solution has identified undiagnosed individuals in eight different diseases and conditions. Moreover, our machine learning solution has demonstrated success across EHR and healthcare claims datasets. Our most recent work has just been published!
Leveraging Machine Learning to Identify Undiagnosed Individuals
Precision screening for familial hypercholesterolemia using electronic health encounter data
This study aimed to accelerate early diagnosis and timely intervention for more than 1.3 million undiagnosed individuals with familial hypercholesterolemia (FH). The Family Heart Foundation led the development and implementation of a machine learning model that was applied to the Family Heart Database™ as well as EHR data from several integrated delivery systems.
Identifying Undiagnosed Individuals with Machine Learning
%
Remain Undiagnosed
Cardiovascular outcomes for people with familial hypercholesterolemia (FH) can be improved with diagnosis and medical management. However, ~70% of individuals with familial hypercholesterolaemia remain undiagnosed in the USA.
We trained the FIND FH® machine learning model using deidentified healthcare encounter data, including procedure and diagnostic codes, prescriptions, and laboratory findings
- The Find FH® model utilized a measured precision (positive predictive value) of 0.85, recall (sensitivity) of 0.45, area under the precision–recall curve of 0.55, and area under the receiver operating characteristic curve of 0.89
- We flagged 1,331,759 of 170,416,201 patients in the national database and 866 of 173,733 individuals in the healthcare delivery system dataset as likely to have familial hypercholesterolemia.
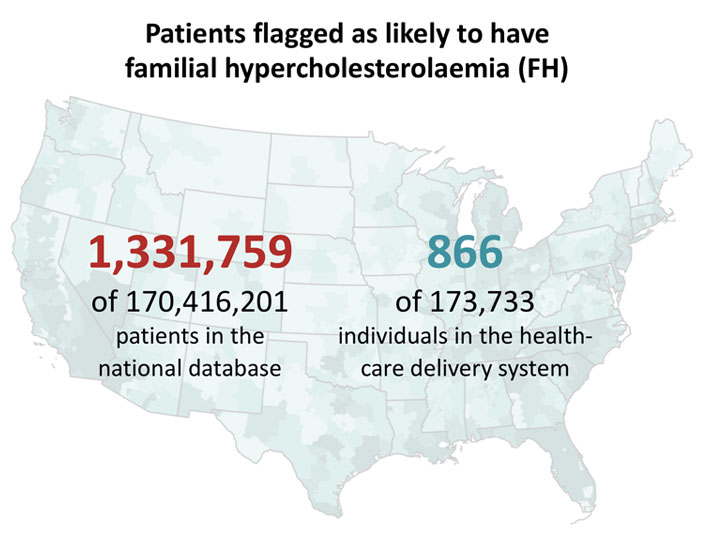
Evidence shows that untreated FH leads to premature atherosclerotic cardiovascular disease (ASCVD). 30% of women under the age of 60 and 50% of men under the age of 50 will have early heart attacks and strokes.
%
Increase in Women
%
Increase in Men
Clinical Experts in the diagnosis of FH reviewed a sample of flagged individuals and applied clinical familial hypercholesterolemia diagnostic criteria.
- Of those reviewed, 87% in the national database and 77% in the healthcare delivery system dataset were categorized as having a high enough clinical suspicion of familial hypercholesterolemia to warrant guideline-based clinical evaluation and treatment.
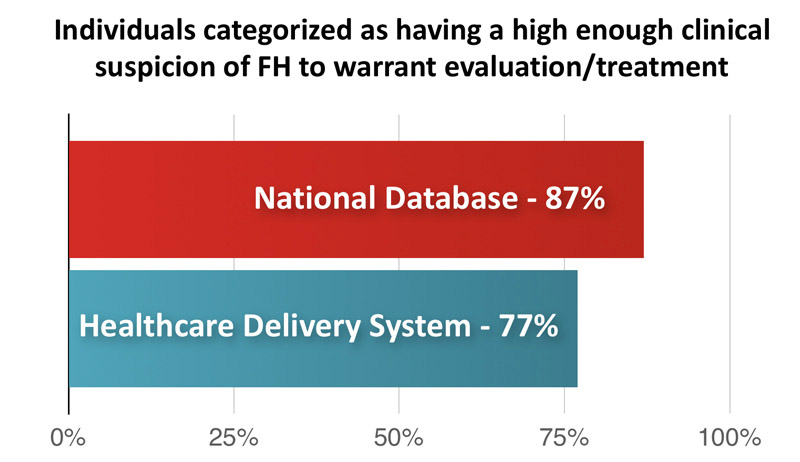
Identifying and Engaging High-Need Populations
Atomo's machine learning technology finds the critical, hidden patterns in digital healthcare records prior to patient diagnoses. Applying these learned patterns to the records of 200+ million patients identifies the patients with the highest unmet need.
- Undiagnosed Individuals
Atomo patient-finding technology has successfully identified undiagnosed patients for >8 diseases/conditions. - Diagnosed and High-Risk Individuals
Atomo methods find those patients at the highest risk and with the most to gain from appropriate treatment. - Segmenting, Prioritizing, and Targeting
Undiagnosed patients are grouped at different levels - to individual physicians, to geographic areas, etc - to facilitate efficient and HIPAA-compliant engagement.
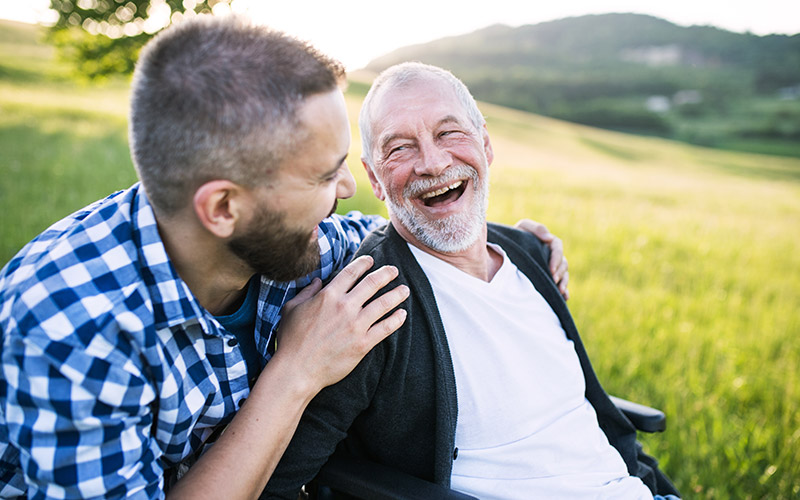
Real World Examples
Finding Undiagnosed Familial Hypercholesterolemia (FH) patients
The Atomo team worked with Stanford and the Family Heart Foundation to find undiagnosed FH patients in Stanford's health network. (published in Nature Digital Medicine) Read Paper Read Press Release
Identifying undiagnosed FH patients across the US population: the FIND FH ® algorithm
The Atomo team, working with the Family Heart Foundation, built a machine learning model to identify undiagnosed FH at the national scale. View Case Study
Media Coverage
Machine learning model for familial hypercholesterolaemia
Using open-source #AI tools to find people with an important, treatable, genetic mutation https://t.co/FgrlhHb27E @LancetDigitalH @TheFHFoundation #FHSummit19 by Kelly Myers and colleagues #openaccess pic.twitter.com/XMQUsc2h7E
— Eric Topol (@EricTopol) October 21, 2019
Great to see! Signs of progress. A #tool to help identify individuals with #FH: Using #DataScience to #FindFH, #KnowFH #TreatHF #CascadeFH @TheFHFoundation
#AI #MachineLearning #BigData #heartdisease. #FHSummit19 @KAWilemon @KellyDMyers @joshuawknowles https://t.co/3IUS6mujKC— George Mensah, MD (@NHLBI_Translate) October 21, 2019
Precision screening for familial hypercholesterolaemia: a machine learning study applied to electronic health encounter data
Familial hypercholesterolaemia: evolving knowledge for designing adaptive models of care
Balanced Dysfunction in the Healthcare Ecosystem Harms Patients
Find FH® machine learning model flags individuals with FH for first time at national level
AI system successfully identifies patients with FH
FIND FH® Machine Learning Model Identifies Individuals With Familial Hypercholesterolemia (FH) For First Time At A National Level
AI can potentially expedite diagnosis, treatment of patients with FH
AI is used to mine health data to find undiagnosed patients, offering a model for other diseases
FIND FH® Makes Identifying and Diagnosing Individuals at Genetic Risk for Early Heart Attacks and Stroke Possible
Machine learning model flags individuals with familial hypercholesterolemia for first time at national level

Reimagine Better Solutions
Atomo can help you leverage technology and big data to solve your most difficult healthcare challenges